Prescriptive Quality Analytics Service
Prescriptive Analytics for Quality Optimisation
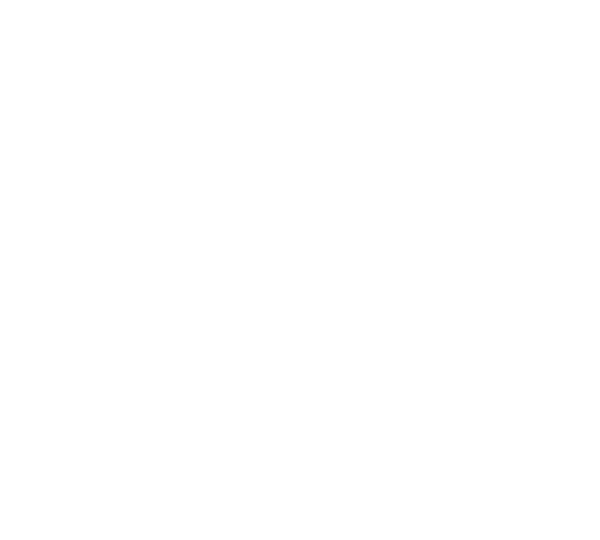
The PREVENTION (Prescriptive Analytics for quality optimization) component addresses the prescriptive quality analytics needs of COALA.
PREVENTION aims at building predictive quality models and prescribing mitigating actions to optimize quality-related manufacturing performance indicators. To achieve its aims, PREVENTION utilizes 3 types of analytics: descriptive, predictive, and prescriptive analytics.
PREVENTION aims at covering the whole lifecycle of data analytics in order to derive insights on quality while enabling the development of several independent analytics processes with different scopes (descriptive, predictive, prescriptive) from multiple data sources and algorithms.
Main features of COALA Prescriptive Quality Analytics Service:
Exploratory Analytics
Statistics for the quality of the production as a whole and for its individual components, i.e. products and defects
Risk Assessment
Evaluation of the criticality of the defect with respect to the products and parts affected
Predictive Quality
Predictions about product defects and defects in the upcoming days
Quality Recommendations
Recommendations for actions in quality control
DIA Core system design focuses on five elements:
Subjective satisfaction
users find the system pleasant and appropriate
Ease of appropriation
users can remember easily how to use the system
Reliability
users cannot make too many or too severe errors and can recover easily
Ease of learning
new users can achieve their goals easily
Efficiency
users can perform tasks quickly through an easy process
Videos
Scientific Publications
M. Fikardos, K. Lepenioti, A. Bousdekis, D. Apostolou, G. Mentzas & E. Bosani. (2022). An Automated Machine Learning Framework for Predictive Analytics in Quality Control. International Conference Advances in Production Management Systems (APMS 2022).
A. Bousdekis, K. Lepenioti, D. Apostolou & G. Mentzas. (2022). Data Analytics in Quality 4.0: Literature Review and Future Research Directions. International Journal of Computer Integrated Manufacturing.