INDUSTRY SCENARIO – WHITE GOODS
Human-AI collaboration in quality control of White Goods
COALA Assistant supporting quality control at the ZHT dedicated laboratory at the MWO Factory in Cassinetta, Italy © Whirlpool
Objectives and Background
Adopt a predictive quality strategy
link the quality control of the finished product with design stage and shop floor
reconfigure its production line and facilitate root cause analysis
The Whirlpool use case is focused on the implementation of the COALA Digital Intelligent Assistant (DIA) to support the activity in the Zero Hour Testing (ZHT) laboratory of the Microwave and Oven (MWO) factory.
The ZHT is a statistical quality check on functional and aesthetical aspects applied in a dedicated laboratory environment out of production flow on some finished products (MWO).
Currently, the procedure is executed manually by a Laboratory Operator and it is fixed, statically defined during the process design phase both for what concerns checklist and reference parameters, and for statistical product withdrawal rate.
This ZHT procedure is then executed in the mass production phase of the production lifecycle as a prescriptive list of elements that is rarely modified, generally only in case of relevant quality issues triggered by KPI monitoring and control alerts or by relevant market issues.
In any case this review is, generally, not re-looping the product design process, limiting the reaction scope on mitigation actions at production level and reducing the possibility to anticipate similar quality issues for other products in development.
This approach, not guaranteeing the identification of all the defected products exiting from production lines, leads also to products that experience No-Fault-Found (NFF) effects in the warranty period. This late identification during usage leads to increasing costs of warranty policies and customer dissatisfaction.
Moreover, the quality control at the very last stage of the production process constitutes mainly a reactive approach aiming at averting the delivery of defected products to customers and at correcting inefficiencies in the production process for the next batches.
Expected Impacts
Business Scenarios #1:
AI-assisted Quality Control in ZHT Lab – for ZHT Operator
AS-IS situation:
• ZHT Operators executes the quality control based on his own experience and memory, referring to a pre-defined and statical quality checklist. At any new product introduction a dedicated training has to be setup.
• The information required during the SOP has to be retrieved manually from various information systems and defects are inserted manually.
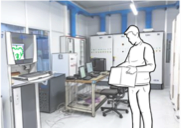
TO-BE situation
• The COALA solution will allow ZHT operators to be driven into checklist execution and supporting to easily retrieve the requested information about products and quality, leveraging on recommendation driven by predictive analytics.
• The COALA Digital Intelligent Assistant will replace current manual digital input of identified defects into legacy systems, leveraging on vocal control.
• COALA will support operator’s skills and competencies development, adapting checklist level of details to the real information needs and helping learning phase from novice to expert.
• The COALA solution will enable the possibility to capture operator’s knowledge and experience, making it available to Whirlpool quality organization to boost cross-functional improvement actions (production and product development).
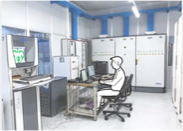
Business Scenarios #2:
AI-assisted Quality Control in ZHT Lab – for ZHT Lab Supervisor
AS-IS situation
• ZHT lab supervisor gets access to ZHT operator activity through legacy systems data and through the informal dialogues at the end of the shift. At the beginning of the shift he passes the key attention points to be addressed during the working time (expected products to be controlled).
• The access to quality data in legacy system has to be done manually with high complexity in cross functional analysis
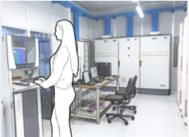
TO-BE situation
• The COALA solution will allow ZHT lab supervisor to strictly monitor quality control process and drive to higher effectiveness through the recommendations resulting by predictive quality approach (quality risk assessment and checklist management) . He will be able also to monitor the effectiveness of quality control suggestions and of the resulting improvement actions through the impact evaluation on quality KPIs.
• Lab supervisor will be facilitated in managing operators skills and competencies development with faster and more effective learning process.
• COALA solution will provide also an effective tool supporting root cause analysis, and the possibility to capture and capitalize the knowledge and expertise of ZHT laboratory to support quality continuous improvement process.
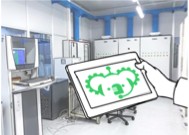